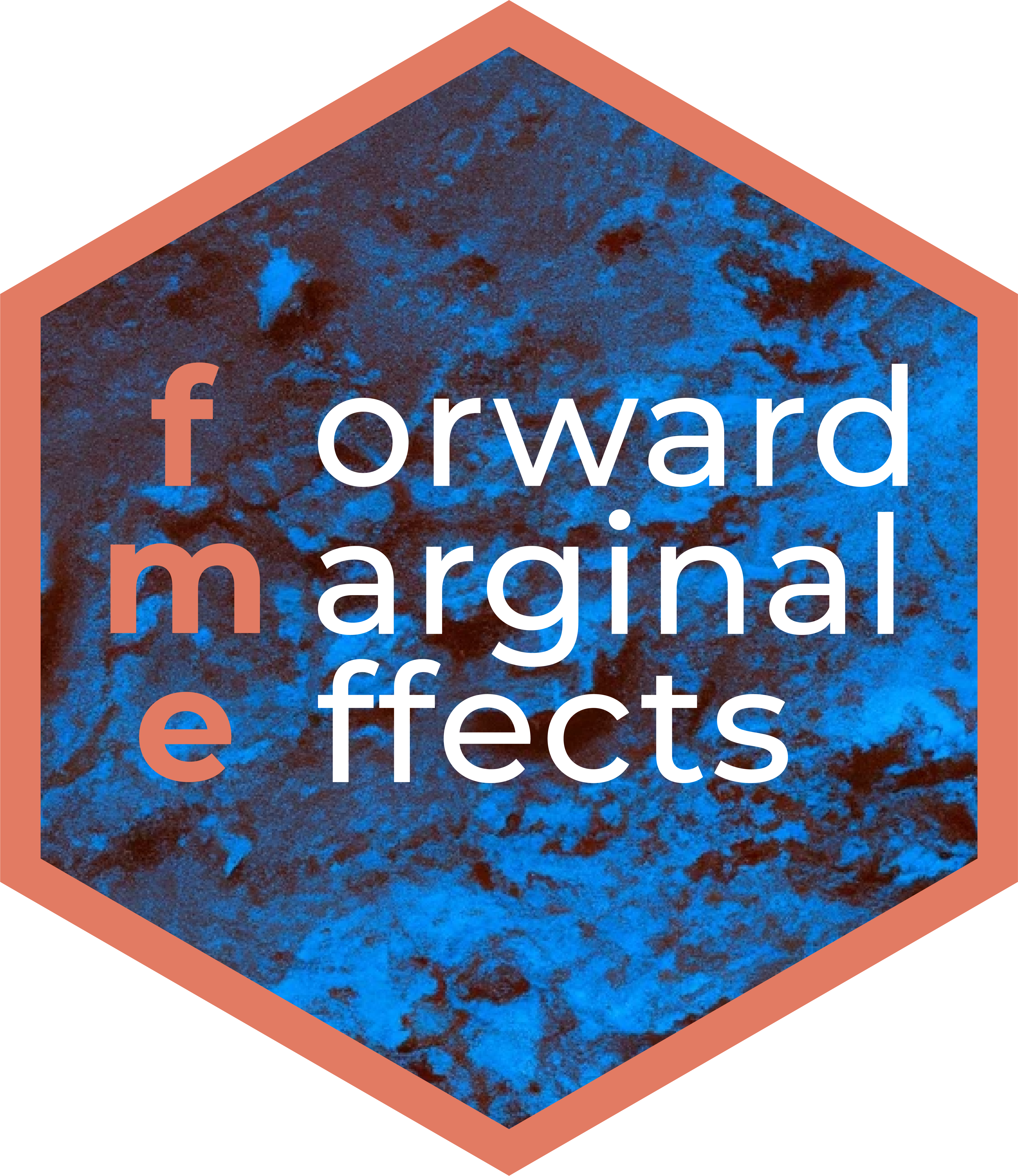
R6 Class computing Average Marginal Effects (AME) based on Forward Marginal Effects (FME) for a model
Source:R/ame.R
AverageMarginalEffects.Rd
The AME is a simple mean FME and computed w.r.t. a feature variable and a model.
Public fields
predictor
Predictor
objectfeatures
vector of features for which AMEs should be computed
ep.method
string specifying extrapolation detection method
results
data.table with AMEs computed
computed
logical specifying if compute() has been run
Methods
Method new()
Create a new AME object.
Usage
AverageMarginalEffects$new(model, data, features = NULL, ep.method = "none")
Arguments
model
The (trained) model, with the ability to predict on new data. This must be a
train.formula
(tidymodels
),Learner
(mlr3
),train
(caret
),lm
orglm
object.data
The data used for computing AMEs, must be data.frame or data.table.
features
If not NULL, a named list of the names of the feature variables for which AMEs should be computed, together with the desired step sizes. For numeric features, the step size must be a single number. For categorial features, the step size must be a character vector of category names that is a subset of the levels of the factor variable.
ep.method
String specifying the method used for extrapolation detection. One of
"none"
or"envelope"
. Defaults to"none"
.
Examples
# Train a model:
library(mlr3verse)
library(ranger)
set.seed(123)
data(bikes, package = "fmeffects")
task = as_task_regr(x = bikes, id = "bikes", target = "count")
forest = lrn("regr.ranger")$train(task)
# Compute AMEs for all features:
\dontrun{
overview = AverageMarginalEffects$new(
model = forest,
data = bikes)$compute()
summary(overview)
# Compute AMEs for a subset of features with non-default step.sizes:
overview = AverageMarginalEffects$new(model = forest,
data = bikes,
features = list(humidity = 0.1,
weather = c("clear", "rain")))$compute()
summary(overview)
}
Examples
## ------------------------------------------------
## Method `AverageMarginalEffects$new`
## ------------------------------------------------
# Train a model:
library(mlr3verse)
#> Loading required package: mlr3
library(ranger)
set.seed(123)
data(bikes, package = "fmeffects")
task = as_task_regr(x = bikes, id = "bikes", target = "count")
forest = lrn("regr.ranger")$train(task)
# Compute AMEs for all features:
if (FALSE) { # \dontrun{
overview = AverageMarginalEffects$new(
model = forest,
data = bikes)$compute()
summary(overview)
# Compute AMEs for a subset of features with non-default step.sizes:
overview = AverageMarginalEffects$new(model = forest,
data = bikes,
features = list(humidity = 0.1,
weather = c("clear", "rain")))$compute()
summary(overview)
} # }
## ------------------------------------------------
## Method `AverageMarginalEffects$compute`
## ------------------------------------------------
# Compute results:
if (FALSE) { # \dontrun{
overview$compute()
} # }